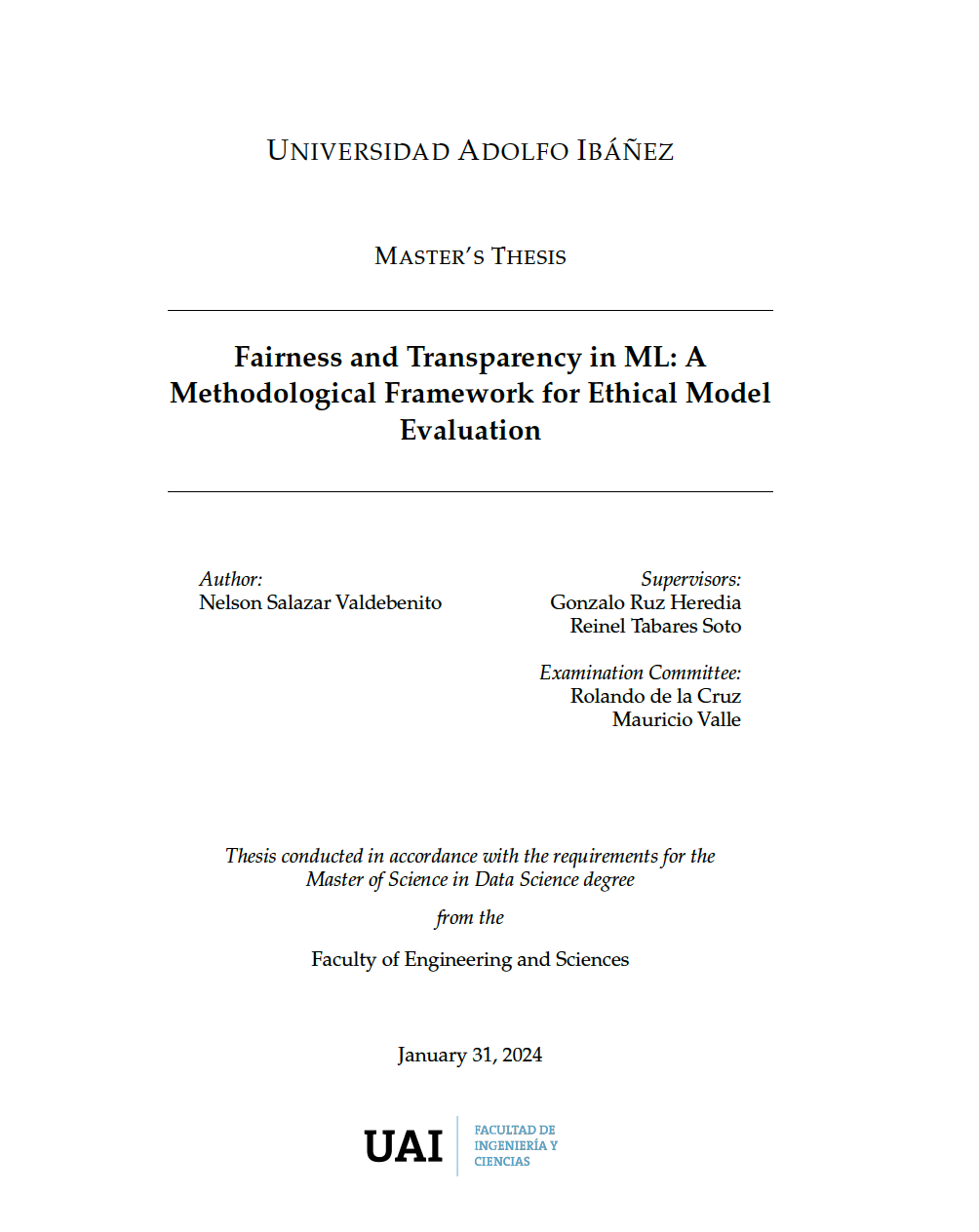
In the times when AI and ML applications are more widely spread across our society, new challenges arise when these are being used by public organisations. Ethical concerns may emerge for various reasons, but especially when these models inherit certain biases that could eventually lead up to hurting and/or punishing people with no reason whatsoever. In this study, a comprehensive methodology is introduced to address ethical considerations in machine learning, particularly focusing on biases and disparities. This thesis showcases the application of this methodology through two experiments, each involving two models: a base model without ethical considerations and an improved one. The primary objective was to evaluate and mitigate inequalities and biases in machine learning models, in this case, using data from Chile’s Public Criminal Defence Office (DPP), which needed a tool that could help in predicting the outcome of a criminal trial. The results were promising, with improvements observed in both models. Specifically, for one of our experiments, there was a reduction in the false negative rate from 40.54% to 31.63%. Additionally, disparities between attributes were reduced by an average of 44.37%. The methodology not only aids in understanding these ethical challenges but also offers tools to optimize and address them, all while maintaining performance.
Nelson Salazar Valdebenito's Master's thesis, "Fairness and Transparency in ML: A Methodological Framework for Ethical Model Evaluation," addresses the ethical challenges in machine learning, specifically focusing on biases and disparities.
Abstract: In the times when AI and ML applications are more widely spread across our society, new challenges arise when these are being used by public organisations. Ethical concerns may emerge for various reasons, but especially when these models inherit certain biases that could eventually lead up to hurting and/or punishing people with no reason whatsoever. In this study, a comprehensive methodology is introduced to address ethical considerations in machine learning, particularly focusing on biases and disparities. This thesis showcases the application of this methodology through two experiments, each involving two models: a base model without ethical considerations and an improved one. The primary objective was to evaluate and mitigate inequalities and biases in machine learning models, in this case, using data from Chile’s Public Criminal Defence Office (DPP), which needed a tool that could help in predicting the outcome of a criminal trial. The results were promising, with improvements observed in both models. Specifically, for one of our experiments, there was a reduction in the false negative rate from 40.54% to 31.63%. Additionally, disparities between attributes were reduced by an average of 44.37%. The methodology not only aids in understanding these ethical challenges but also offers tools to optimize and address them, all while maintaining performance.
This thesis is framed within Technical Cooperation Agreement No. ATN/ME-18240-CH, between BID Lab and Universidad Adolfo Ibáñez.
This thesis was completed in fulfillment of the requirements for the Master of Science in Data Science degree from the Faculty of Engineering and Sciences at Universidad Adolfo Ibáñez. The author, Nelson Salazar Valdebenito, was supervised by Gonzalo Ruz Heredia and Reinel Tabares Soto, with Rolando de la Cruz and Mauricio Valle serving on the examination committee. The thesis was successfully defended on January 31, 2024.